2023.11.17
제주도 공모전이 아직 끝나지 않았지만 대구 공모전 데이터도 보면서
리더보드에 점수도 남기고..
그 기념으로 코딩이야기 두 번째, 타이타닉 생존자 예측 실습을 "내 방식대로"
다시 한 번 정리해보기로 했다.
글의 순서는
1. 타이타닉 데이터셋 살펴보기
2. Feature Engineering
3. Pipeline 구축
import numpy as np
import pandas as pd
import matplotlib.pyplot as plt
import seaborn as sns
plt.rcParams = "Malgun Gothic"
titanic = pd.read_csv('titanic.csv')
titanic
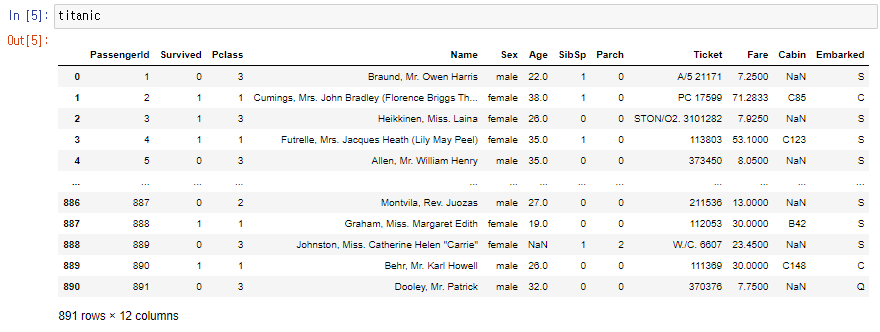
우리가 예측할 target column은 Survived
1 : 생존 / 0 : 사망
즉, 이진분류 문제이다.
titanic.info()
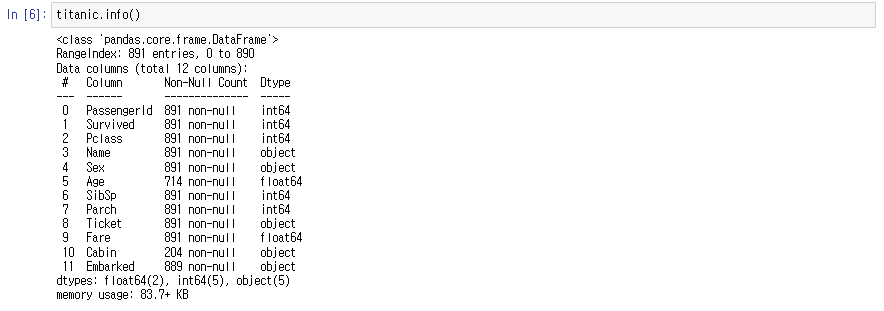
null값 처리를 해야 하는 column이 세 개 ( Age, Cabin, Embarked )
titanic.nunique()
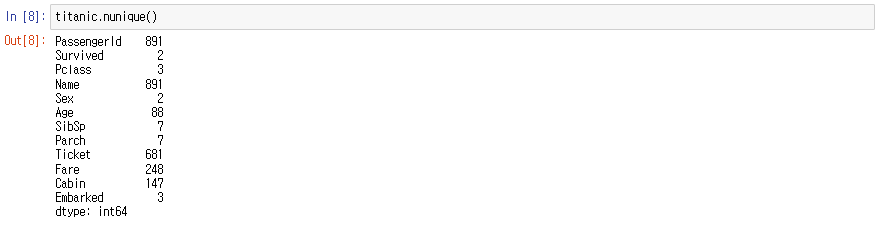
2. Feature Engineering
# Age column은 평균값으로, Embarked column은 최빈값으로 대체
titanic.Age.fillna(titanic.Age.mean(), inplace = True)
titanic.Embarked.fillna(titanic.Embarked.mode()[0], inplace = True)
3. Pipeline 구축
pipeline 구축하기에 앞서 먼저 고유값과 column type에 따라 분류해주었다.
# 제외할 columns = ['PassengerId', 'Name', 'Cabin']
numeric_feature = ['Age', 'Fare']
low_cardinality_feature = ['Pclass', 'Sex', 'SibSp', 'Parch', 'Embarked']
high_cardinality_feature = ['Ticket']
사이킷런에 내장되어 있는 train_test_split 메소드를 사용했고
test_size = 0.3, random_state = 0으로 고정한 후 데이터를 학습 / 테스트로 분리했다.
from sklearn.model_selection import train_test_split
X_train, X_test, y_train, y_test = train_test_split(titanic[numeric_feature + low_cardinality_feature + high_cardinality_feature],
titanic['Survived'], test_size=0.3, random_state=0)
본격적으로 Pipeline을 구축해주었다.
고유값이 적은 column은 OneHot Encoder 적용
고유값이 많은 column은 Target Encoder 적용
numeric feature는 그대로 사용
Model은 LGBMClassifier로 설정해서 구축해주었다.
from sklearn.pipeline import Pipeline
from lightgbm import LGBMClassifier
from sklearn.pipeline import make_pipeline
from sklearn.preprocessing import OneHotEncoder
from category_encoders import TargetEncoder
from sklearn.compose import ColumnTransformer
from sklearn.preprocessing import FunctionTransformer
low_car_categorical_transformer = Pipeline(
steps=[("encoder", OneHotEncoder(handle_unknown="ignore"))
]
)
high_car_categorical_transformer = Pipeline(
steps=[
("encoder", TargetEncoder())
]
)
column_transformer = ColumnTransformer(
transformers=[
("num", "passthrough", numeric_feature),
("low_car_cat", low_car_categorical_transformer, low_cardinality_feature),
("high_car_cat", high_car_categorical_transformer, high_cardinality_feature)
]
)
preprocessor = Pipeline(
steps=[
("column", column_transformer)
]
)
model = Pipeline(
steps=[
("preprocessor", preprocessor),
("regressor", LGBMClassifier(verbose = -1))
]
)
이 후 점수 검증을 위해 optuna 패키지를 import 했고
LGBMClassifier 최적의 하이퍼파라미터를 Hyper Optuna 기법으로 찾아냈다.
평가 방식은 정확도를 뜻하는 accuracy를 사용했고
sampler의 seed도 0으로 고정했다.
import optuna
param_distributions = {
'regressor__learning_rate': optuna.distributions.FloatDistribution(0.01, 0.5), # 학습률
'regressor__n_estimators': optuna.distributions.IntDistribution(100, 1000), # 트리의 개수
'regressor__max_depth': optuna.distributions.IntDistribution(3, 20) # 트리의 최대 깊이
}
optuna_search = optuna.integration.OptunaSearchCV(model, param_distributions, cv=5, scoring='accuracy', n_trials=50,
study=optuna.create_study(sampler=optuna.samplers.TPESampler(seed=0), direction="maximize"))
optuna_search.fit(X_train, y_train)
Best Parameter와 Best Score는 아래과 같다.

시각화도 곁들여서 분석하면 더 좋은 결과가 나올 것이다.
하지만 오늘 분석은 여기서 끝
'코딩이야기' 카테고리의 다른 글
하루하루 코딩이야기 4 : XGBoost 실습 - 위스콘신 유방암 예측 (0) | 2023.12.01 |
---|---|
하루하루 코딩이야기 3 : pd.to_datetime(), Datetime feature 다루기 (0) | 2023.11.23 |
하루하루 코딩 이야기 1 : Iris 붓꽃 품종 예측하기 (1) | 2023.11.14 |